Cost Effective AIGC Network Solution by Asterfusion ROCEv2 Ready Switch
written by Asterfuison
Table of Contents
In the year 2023, we witnessed a remarkable surge in the advancements of artificial intelligence technologies, particularly represented by AIGC’s large-scale models like ChatGPT and GPT-4. Without a reliable network infrastructure, the training of such large-scale models would be an insurmountable challenge. To successfully establish extensive training model clusters, we require not only powerful GPU servers and network cards but also a robust network infrastructure. It is worth exploring what type of powerful network is required to support the seamless operation of AIGC? Moreover, is there a more cost-effective alternative available?
What is AIGC Technology?
AIGC stands for AI-Generated Content. Its main focus is to utilize AI for automatically generating content. However, on a broader scale, AIGC represents the progress of AI from perception and understanding to creation and generation. This new trend in AI technology goes beyond the traditional analytical capabilities of AI and now involves the automatic generation of various forms of content such as text, images, music, video, and interactive 3D content. The advancement in AIGC is achieved through extensive training data and algorithmic models.[1]
What is AIGC Network Workload?
Whether in Natural Language Processing (NLP), Machine Learning (ML), Fin-tech or Autonomous Driving, a common feature of AI workloads is that they are data and compute intensive.
A typical AI training workload involves billions of parameters and large sparse matrix computations spread across hundreds or thousands of processors (CPUs, GPUs, or TPUs.) These processors perform intensive computations and then exchange data with peer processors. Data from peer processors is merged with local data and a new round of processing begins.
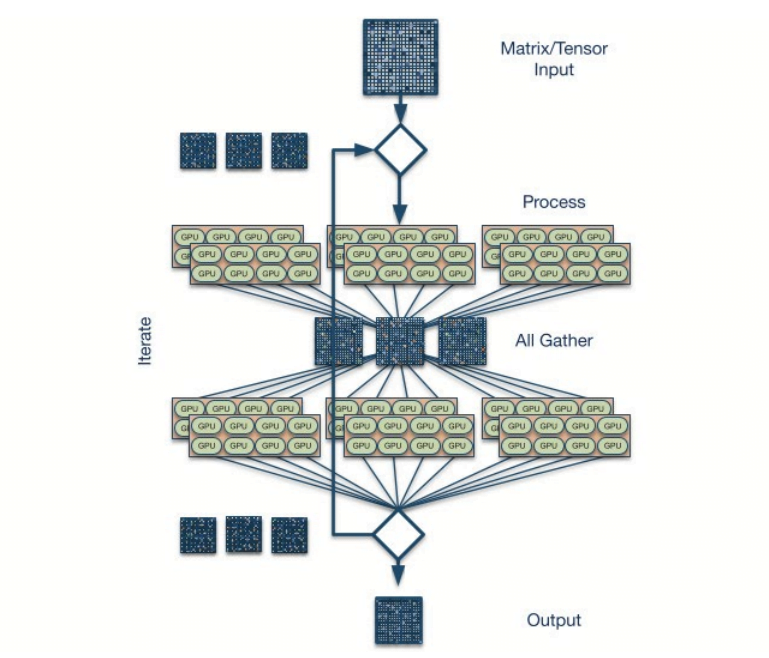
In this compute-exchange-reduce cycle, about 20-50% of the operation time is spent communicating across the network, so network bottlenecks have a significant impact on computation time.
What is AIGC Data Center Networking?
AI data center networking refers to the networking infrastructure and technologies used to support the efficient and high-performance operation of artificial intelligence (AI) workloads within data centers. The goal of the AIGC network is to increase the utilization of GPU servers, and to do so, there are stringent requirements for network scalability, communication latency and reliability. Here are some key aspects of AIGC network:
High-speed Networking: InfiniBand or Ethernet?
InfiniBand is a common networking technology used in high performance computing (HPC). But as we mentioned, AI/ML workloads are network-intensive, unlike legacy HPC workloads. Compared to InfiniBand, Ethernet is considered as an open alternative solution for AI networking due to its scalability, interoperability, cost-effectiveness, flexibility and open ecology. With technologies such as RoCE and lossless Ethernet (e.g., ECN, PFC), as well as high-performance switches, Ethernet-based cluster networks can also provide good support for high-speed networking.
Network Fabric and Scalability:More Expansion, More Complexity?
AI data center networks can be designed using a variety of fabrics, mostly using any-to-any non-blocking Clos fabrics to optimize LLM-training. Modern AI applications require large clusters equipped with thousands of GPUs and storage, and these clusters must scale up as demand grows. However, as shown in Figure 2, this results in high network cost and complexity.
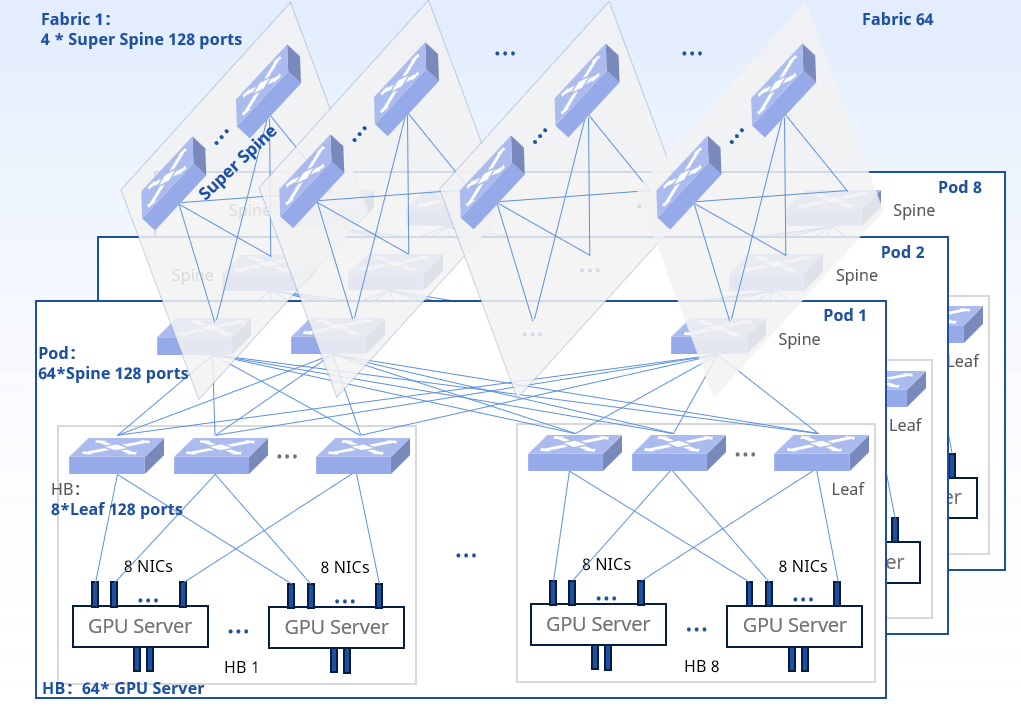
Low-cost AIGC Network Solution Built with Asterfusion 400G Ethernet Switches
With GPU speeds doubling every other year, avoiding compute and network bottlenecks is becoming much more critical – we need a more scalable and cost-effective AIGC network design.
A rail is the set of GPUs with the same rank on different HB(High-Bandwidth)domains, interconnected with a “rail switch”. Unlike the any-to-any nonblocking Clos Fabric, rail-only network is a simplified architecture that removes network connectivity across GPUs with different ranks in different rails, without compressing performance. Since in LLM training, communication across HB domains occurs only between pairs of GPUs of the same rank in their respective HB domains, redundant links just add unnecessary complexity and cost.
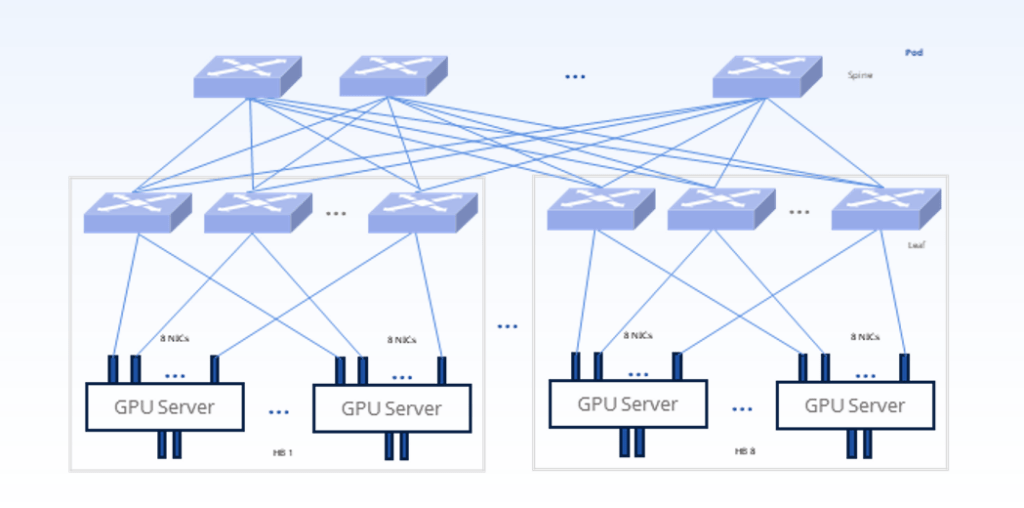
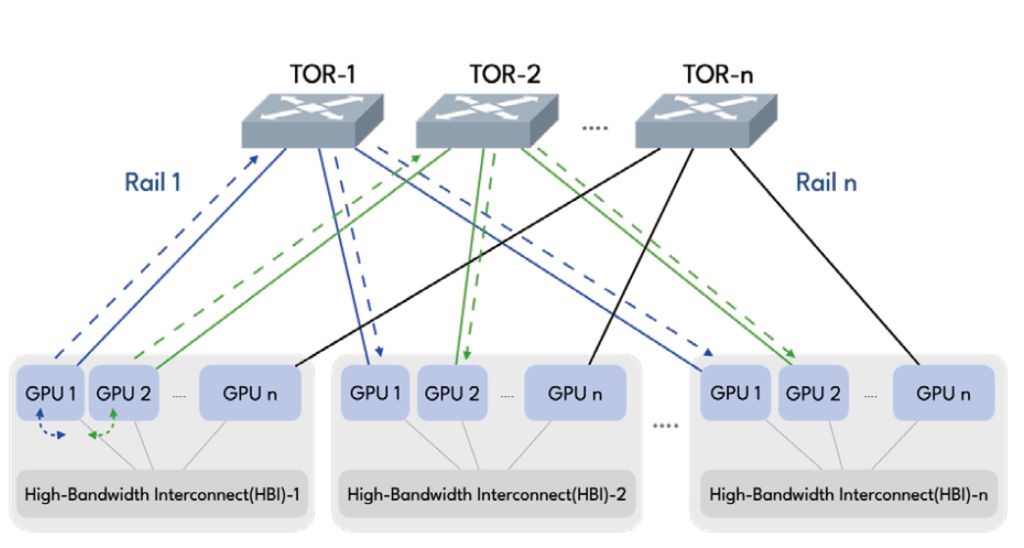
Compared to a conventional rail-optimized GPU cluster (Any-to-Any,400Gbps), with the “rail-only” architecture, the total number of switches needed for a 32,000 LLM-Training GPU cluster is 256 vs 1280 in the state-of-the-art any-to-any CLOS architecture, resulting a 75% cost reduction.[2]
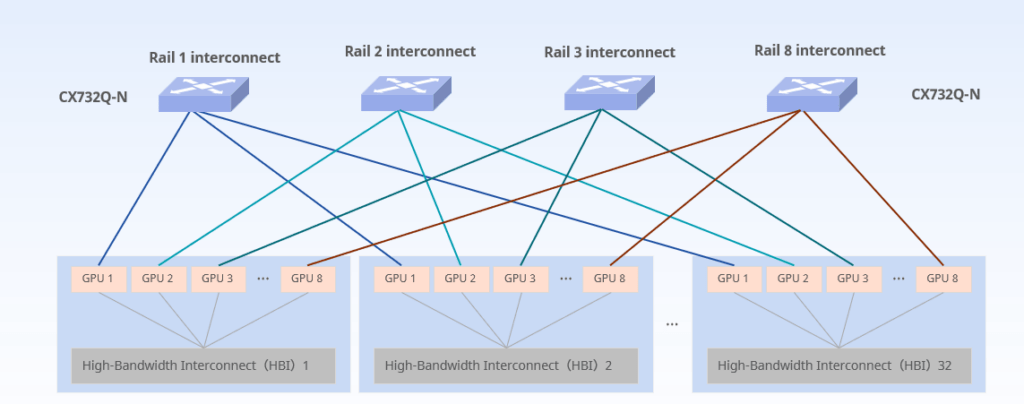
The Asterfusion CX732Q-N comes pre-installed with the enterprise-grade SONiC distribution, a feature set that gives it superior functionality beyond the community version. In addition, the switch is powered by Marvell Teralynx 7 for unrivalled low-latency capabilities. The switch achieves superior performance with a minimalist and energy-efficient philosophy. What can’t be overlooked: this machine offers the most cost-effective 32*400G switch price on the market!
For more details: https://cloudswit.ch/blogs/asterfusion-sonic-32-400g-data-center-switch/
Urgent & Massive Need For 400G/800G Data Center Switches -For AIGC
Data centers are in massive and urgent need of 400G/800G switches to handle the increasing demands of AIGC workloads. As these workloads require high-speed data transmission, network switches with ports transitioning from 100G/200G to 400G/800G are essential. According to a Dell’Oro report, the market will see a significant rise in switches with speeds of 400Gbps and above, accounting for about 70% by 2027.
Excitingly, Asterfusion is set to release our ultra-low latency Ethernet switch with a remarkable speed of 800G (51.2T). Stay tuned for this groundbreaking technology.
-
64-Port 200G QSFP56 Low Latency Data Center Switch, Enterprise SONiC Ready, Marvell Teralynx: CX664P-N
-
32-Port 400G QSFP-DD Low Latency AI/ML/Data Center Switch, Enterprise SONiC Ready,CX732P-N
-
64-Port QSFP28 Low Latency Data Center Switch, Enterprise SONiC Ready, Marvell Teralynx: CX564P-N
-
32-Port QSFP28 Low Latency Data Center Switch, Enterprise SONiC Ready, Marvell Teralynx: CX532P-N
For more, please email bd@cloudswit.ch, or visit our website https://cloudswit.ch/
Reference:
1.AIGC Development Trend Report 2023 (Tencent Research Institute)
2.Weiyang Wang, et al. (2023)How to Build Low-cost Networks for Large Language Models (without Sacrificing Performance)[https://arxiv.org/abs/2307.12169]